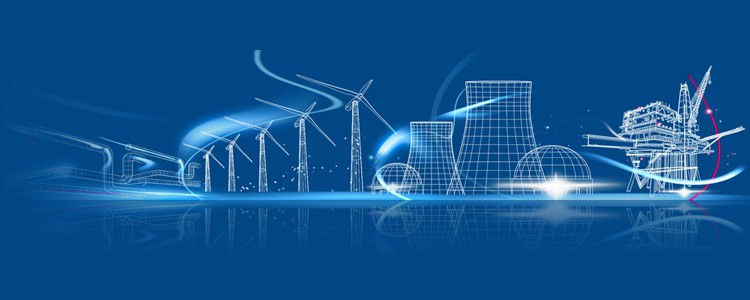
Artificial intelligence is a broad term that is used more and more often to describe sophisticated tech. There is no single widely accepted definition of AI because the concept of it is very complex, new, and continuously developing.
The utilization of steam power to automate production guided the world into the industrial age by launching the first of several industrial revolutions. However, electricity utilization has significantly changed the world and enabled an era of mass production, fostering revolutionary advances in transport, telecommunications, and manufacturing, now called the Second Industrial Revolution. The Third Industrial Revolution started in the digital age, widespread computer use, and, in particular, the Internet and the widespread use of computer technology to automate production.
However, another profound change follows - the fourth industrial revolution whose digital change of the world in terms of speed, scope, and impact on systems should be unique from past encounters. It will be portrayed by scientific and technical breakthroughs that will strongly change existing industries and, along these lines, influence the advancement of society. One of its key drivers is artificial intelligence (AI).
Defining what and how AI will function additionally depends on its end application. In principle, AI enables the automation of processes and activities performed by people today, positively affecting execution, productivity, and proficiency. In doing such, AI is heavily dependent on data. Specifically, from one viewpoint, AI utilizes structured data, such as those from machine sensors, and then again unstructured data, such as manuals, maintenance records, climate, market, and business data, to empower advanced decision-making. To master this effectively, AI utilizes machine discovery that envelops a few ideas, including characteristic language processing, profundity learning, and neural organizations. Machine learning (ML) is presently the most utilized piece of AI, and it is tied in with examining PC algorithms guided by data that are automatically improved by experience.
In recent times, another type of AI is being created, and that is automated machine learning. It is the ultimate process of automated machine learning application to real-world problems, such as data preparation, engineering features, and model selection. It is also increasingly interesting in energetics since it has been seen that there is an issue with organizations lacking expertise in the application of machine learning methods. Many energy specialists point out that maybe the best advantage of AI will be its capacity to effectively exploit huge amounts of operational data when energy is battling various distinctive huge business impacts, including decarbonization, decentralization, and digitization.
Some energy and IT experts state that today’s AI tools offer the best potential to take care of certain problems utilizing, for example, images, such as applications, to facilitate the time-consuming and tedious review measure. In energy, each facility or plant must be inspected, and the measure of such activities that can be computerized utilizing AI is extremely large. In large time series. Such data is examined to foresee future conduct, maintenance needs, or potential impending hazards, so understanding these patterns is critical. It is normal that the fruitful implementation of AI around there could fundamentally decrease energy costs, assisting with taking out various bottlenecks.
In spite of the fact that there are numerous particular cases in energy organizations where AI or ML (machine learning) alone can be applied, the following huge advance in the application of AI will be to change the focus from watching, for instance, the exhibition of a production facility or plant to the presentation of the production unit, analyzing the activity of the whole power network- from production to consumption. The potential of AI to improve predictive and prescriptive capabilities is especially significant today as creation has gotten essentially more complex because of the large portion of energy production and distribution from solar or wind power plants. In addition, AI can take current simulations to a higher level, e.g., to help utilities with finding unstable network areas and increase the safety of field workers.
A potentially critical part for AI could be later on the integration of the power business, for example, by exploiting low-carbon resources, improving forecasting, and strengthening autonomy. Simulator capabilities could likewise be increased to improve their ability and ultimate network synchronization capabilities.
In practice, some leading energy companies have already started working with AI.
In addition to the oil giants analyzing huge amounts of exploration and propulsion data from large oil fields, the American giant General Electric (GE) has applied neural networks and edge controls in its advanced combustion management systems to optimize distribution fuels for gas and aerodynamic turbines. The result is reduced emissions of nitrogen oxides and carbon monoxide and improved overall performance of these turbines. Also, AI at GE provides production planning advice to help dealers and selected designers understand the capabilities of a day-ahead and real-time combined cycle plant, allowing them to better prepare for commitments and to plan nomination and fuel shipments. At the network level, GE has applied ML in analytics to better link inertia to network operators with known and predictable values such as conventional rotating inertia and load.
However, IT and energy experts also agree that at the moment, for most energy companies, there is still a long way to go before they can be widely used and take advantage of any AI. The development of AI is still in the early stages of implementation, and the biggest challenge is data. Everything that AI does requires both background context data and drive data from the process being analyzed. To obtain data, collaboration with others is necessary in order to obtain real and useful data sets. As such, data becomes more accessible over time, so do models evolve and become smarter. Where AI is currently already being implemented, it is actually parts of larger models of AI and experimental and pilot projects of its application, where various energy actors are testing their capabilities or demonstrating their capabilities. However, from what is happening in practice, it cannot be concluded that this is a real adoption of AI in energy.
The key solution is to truly understand the problems that need to be solved before deciding which method to solve them. Then, once the problems are sufficiently articulated, and it is shown that AI or ML is the optimal solution to a given problem, then they must be scaled in a digital environment with appropriately cleaned, modeled, and orchestrated data. That is why some experts point out that the turning point will come when models of bulk collection and organization of data on the model of networked plants and processes such as AI can be adopted in everyday work operations, and not when only the most sophisticated algorithms are developed.
Insiders point to another problem, and that is that there are relatively few experts in the energy companies themselves who can successfully manage the range of operational data necessary for AI advancement. Thus, in addition to finding partners with the necessary expertise in AI and software, energy companies should perhaps undergo a workforce transformation and develop a culture of collaboration between workers and technology. They should integrate AI into their strategic planning and long-term business plans and understand the opportunities that come with them to successfully reshape the company culture, minimize risk, and reap the most benefits from such technical solutions.
On the other hand, government or professional bodies for digitalization of industry should, in that case, define some standard operational problems in energy and establish a methodology for solving them with appropriate data sets so that AI tool vendors can test their solutions on these selected data sets. This would likely allow both an impartial and technically correct assessment of AI solutions and their capabilities, which is crucial for the industry. At the same time, energy companies would get a better idea of how some AI tools, and data sets work together to solve a specific problem.
留言